Blog
The CxO Series: Tips for implementing generative AI from Accenture
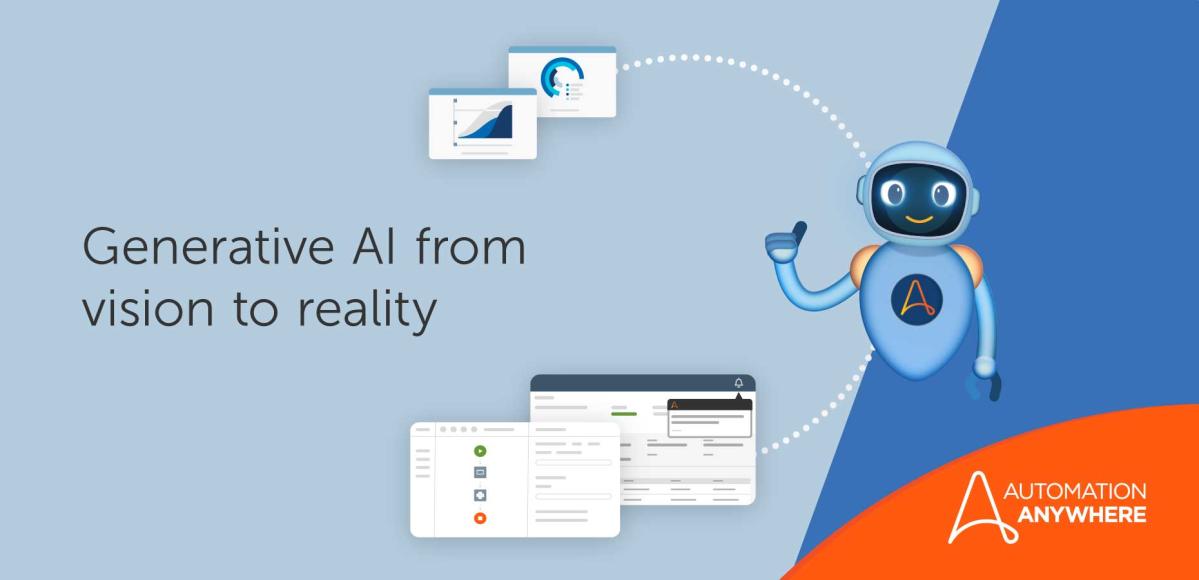
For our latest CxO Series blog, I had the pleasure of speaking with Shallu Gupta, Intelligent Automation CTO for Tech for Operations at Accenture Operations, who drives automation initiatives across operations. We spoke about the rise of generative AI and what trends she’s seeing in implementations among Accenture’s clients.
Can you tell us a little bit about your role and the work you've done leading up to it?
Gupta: I have over 22 years of IT experience and come from a core software development background. My current role involves collaborating with top-tier vendors, delivery teams, and business transformation teams across Accenture to design technology strategies that align with our business objectives. I strive to ensure that our technology roadmap is in sync with our overall business strategy, enabling us to stay competitive in a rapidly evolving technology landscape. Building and managing technology partnerships with key vendors and partners is also a crucial aspect of my role. This ensures that we have access to the latest technologies and solutions that can drive operational efficiency and enhance our business outcomes.
As an Automation CTO, I am committed to staying abreast of emerging technologies, industry trends, and best practices. By fostering a culture of innovation and collaboration, I aim to drive transformative changes that positively impact our business performance.
AI and generative AI are clearly high-priority and top-of-mind topics for your clients. What are some key trends you are seeing and areas where clients are showing the most interest and potential for these technologies?
Gupta: Personally, I am eager to leverage generative AI’s capabilities across all domains within Accenture Operations. At Accenture, we are currently doing multiple experiments across several departments (finance, marketing & research, talent & HR, etc.) along with measuring the impact of generative AI on different phases of the software development life cycle (SDLC), ranging from discovery to deployment as it has the potential to significantly improve developer productivity through code generation, code debugging, and other advanced features.
At our organization, we have already been utilizing AI to deliver intelligent automation solutions to our clients. These solutions encompass various areas such as document digitization, natural language processing (NLP)-based automation, and predictive analysis. By incorporating AI technologies, we have helped our clients reduce costs, enhance operational efficiency, and elevate the overall customer experience.
In the current landscape, clients are increasingly seeking end-to-end integrated solutions rather than individual point solutions. They desire a comprehensive platform that offers a unified ecosystem instead of relying on multiple disjointed tools. The introduction of generative AI has further fueled this trend, with a particular emphasis on NLP and natural language generation (NLG). Clients are keen to leverage these technologies to enhance customer service, automate conversations, and generate high-quality written content that closely resembles human-generated content.
Aligning business goals to an AI strategy should be paramount. What are some of the ways you see that are best to measure success?
Gupta: There would be both quantitative and qualitative measures, such as:
- Customer feedback
- Influence on financial metrics (cost, revenue, ROI)
- Impact on operational metrics (efficiency, effectiveness, productivity)
- Employee satisfaction (work-life balance)
What are some other considerations organizations need to be mindful of when implementing their AI strategy?
Gupta: I believe organizations should be mindful of the following:
- Skill gap analysis
Organizations should conduct a comprehensive skill gap analysis to identify areas where additional training or hiring is required. - Command center
Implementing a command center can greatly benefit organizations when it comes to managing AI systems, as it provides real-time monitoring and oversight of AI operations, performance, and potential issues. - Cost-benefit analysis
It's crucial for organizations to conduct a cost-benefit analysis before implementing AI solutions to ensure they align with their overall business objectives. - Effective bias management
Implementing robust bias management practices involves diverse and representative data sets, regular auditing of algorithms, and continuous monitoring to identify and mitigate biases. This ensures fairness, transparency, and ethical use of AI technologies. - Establish a robust support function
Organizations should establish a strong support function to assist employees and stakeholders in utilizing AI technologies effectively. Having a reliable support system helps overcome challenges, ensures smooth operations, and maximizes the value derived from AI systems. - Proper feedback mechanism
Establishing a feedback mechanism is essential for organizations to continuously improve their AI strategies. This ultimately drives innovation and ensures the alignment of AI solutions with the evolving needs of the organization and its stakeholders.
By considering these additional factors alongside the ones previously listed, organizations can enhance the success and effectiveness of their AI strategies while minimizing risks and maximizing the benefits AI can bring to their operations.
What are some of the key barriers that organizations should watch out for?
Gupta: The most important barrier should be related to data privacy and security and ensuring businesses have a firm grasp on the way data is handled, processed, protected, secured, and used. Other barriers are how organizations are handling copyright issues, generating unbiased output, and consistency.
How can organizations ensure that the strategy they adopt today is sustainable in the long term?
Gupta: There are lots of opportunities we see with the advancement of AI, and organizations are under pressure to adopt it. I would say each organization should think responsibly, as innovation brings in lots of opportunities but responsibilities as well. Here are a few things organizations should consider:
- Identify the areas to first begin the AI journey and plan a long-term strategy on their adoption roadmap.
- Plan for the kinds of skill sets you need and build a strategy around skilling your resources.
- Identify key metrics to measure their success.
- Understand the limitations and the models that you are going to use.
- Put a robust governance model around complying with relevant regulations.
- Put best testing practices and quality checks in place before any AI system is put into production.
- Put in systems to continuously monitor your systems and a trained support model to fix issues immediately and permanently.
- Establish a strong feedback mechanism.
How do you see the generative AI space evolving over the next 12-24 months?
Gupta: The continuous evolution of each layer in the generative AI tech stack indicates promising improvements in its effectiveness for consumers. Moving forward, organizations will have the ability to fine-tune the technology according to their specific needs, leveraging their proprietary data to integrate it seamlessly into their applications. The demand for skills related to fine-tuning existing models based on organizational requirements is expected to soar. I see prompt engineering as likely to become an increasingly important skill for developers and engineers.
The rapid pace of advancements is already giving rise to new models, and we anticipate the availability of even more mature pre-trained models in the market in the next 1-2 years. These models will provide organizations with enhanced capabilities and further options for implementing generative AI solutions that align with their unique business objectives.
Hear insights from more industry experts at Imagine 2023.
Share this:
Related Blogs
About Anoop Sahgal
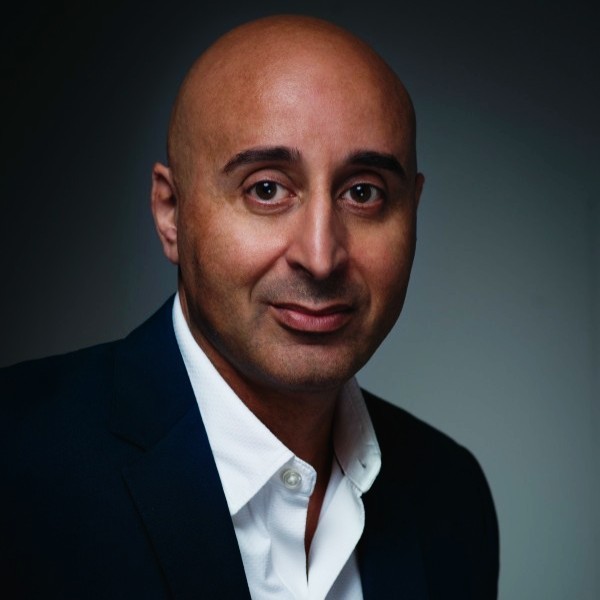